Blog
Featured
Managing Supply Shortages: Lessons Learned from Recent Headlines
Supply chains are the lifeblood of modern businesses, ensuring the smooth flow of goods and services from manufacturers to end consumers. However, recent events like the Baltimore Port Bridge collapse and the Taiwan earthquake have underscored the vulnerability of these intricate networks. As supply chain leaders, we must learn from these disruptions and proactively address their impact.
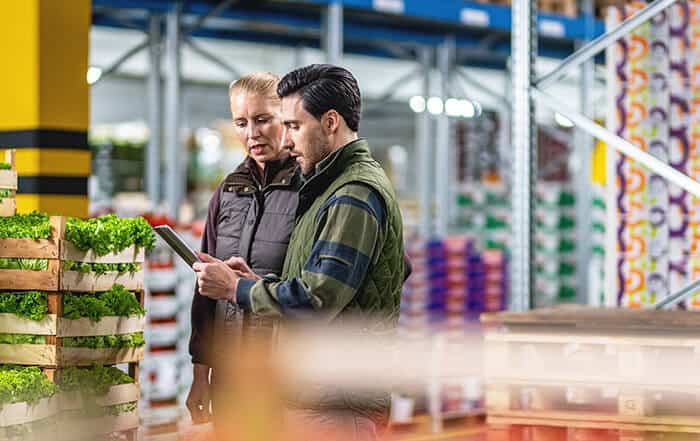