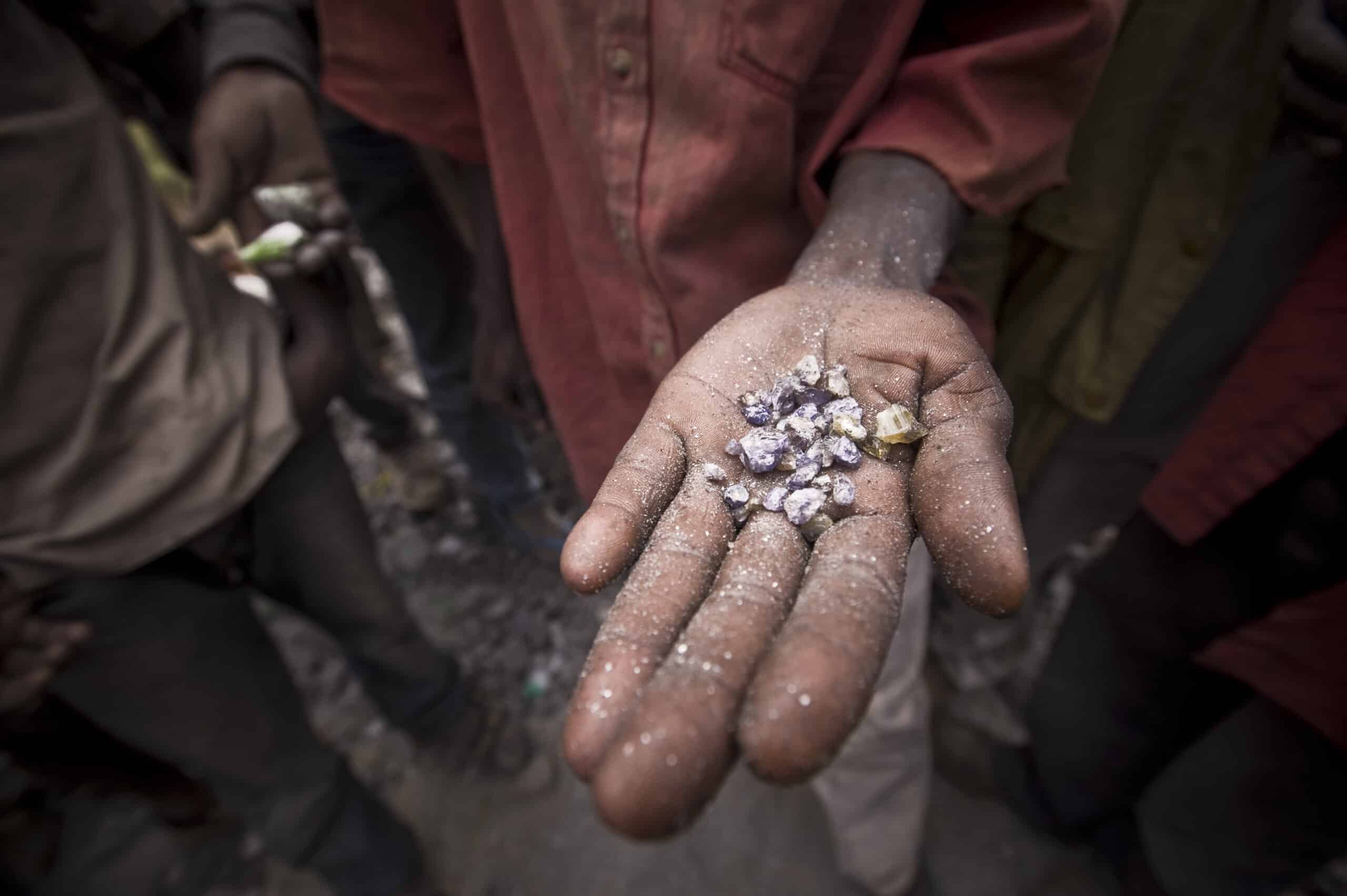
China Rare Earth Export Restrictions: Global Trade Impact
License delays and sensitive data requirements push firms to rethink sourcing strategies. The global economy runs on elements most people have never heard of. Rare earth elements (REEs) power everything from smartphones to wind turbines, yet they remain dangerously concentrated. When China introduced new export restrictions in 2025, businesses dependent on rare earth supply chains...